Researchers at our institute presented two papers at the IEEE International Workshop on Signal Processing Advances in Wireless Communications (SPAWC) in Oulu, Finland:
- Improving Triplet-Based Channel Charting on Distributed Massive MIMO Measurements in Special Session on Machine Learning for Communications
- Adaptive Neural Network-based OFDM Receivers in Special Session on ML for PHY - From Theory to Implementation
Our Contributions to Channel Charting
Massive MIMO systems require high-dimensional channel state information (CSI) that is captured continually in order to estimate the wireless channel between transmitter and receiver. Since the CSI is mainly dependent on the user position, it can be recycled for several tasks such as user positioning. Supervised positioning (“fingerprinting”) relies on accurate ground truth position labels, which are, in practice, not always available. Therefore, a new signal processing pipeline called “Channel Charting” has been introduced, which aims to create a virtual map of the radio environment in a self-supervised manner.
At SPAWC, we presented work which applies state-of-the-art triplet-based Channel Charting to real channel measurements captured by our DICHASUS channel sounder. Since channel charting has mainly been applied to simulated data in the past, we were happy to present competitive results on real data. Furthermore, we investigated the triplet selection process to understand the properties of channel measurement datasets that are desirable for the functioning of Channel Charting. Our comprehensible tutorial on Channel Charting makes it possible for everyone to reproduce our results easily.
Our Contributions to Neural Network-Based OFDM Receivers
Following the general trend of applying machine learning methods in communications, the idea of neural network-based OFDM receivers has been driven forward in recent years. The resulting investigations show competitive performances and gains compared to conventional (filter-based) baseline systems. Training these neural network-based receivers typically aims at achieving the optimal universal performance of the OFDM receiver and a resulting applicability in an ensemble of different scenarios.
At SPAWC, we presented the paper “Adaptive Neural Network-based OFDM Receivers”, which further enhances the concept of neural network-based receivers. We focused on the idea that a receiver optimized for universality does not necessarily provide the best possible performance in any static scenario and that not all characteristics of the channel are captured in the initial training dataset, i.e., unforeseen effects can occur during a transmission. Based on these considerations, we proposed the idea of continuously retraining OFDM receivers online to adapt them to the current channel conditions resulting in a better performance, i.e., a lower bit error rate, compared to static receivers. We show adaptation gains for static channel conditions, e.g., a certain UE velocity, for out-of-specification usage and for temporal alterations, e.g., interference.
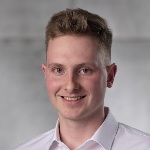
Phillip Stephan, M.Sc.
Research Assistant
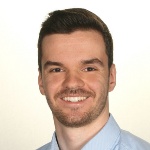
Moritz Fischer, M.Sc.
Research Assistant
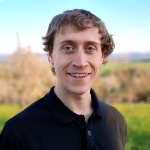
Florian Euchner, M.Sc.
Research Assistant